How Can We Improve the Efficiency of Electricity Pricing Systems?
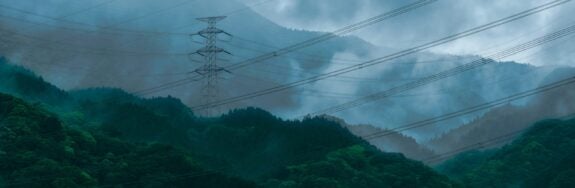
Consumer prices for electricity do not align with generation cost, causing inefficient consumer behavior. Simple time-of-use rates and limited critical-peak pricing can each correct around 10% of this mispricing, but complex rates often backfire.
At a Glance
Key Challenge
Prices charged by electric utilities rarely match the current price of electricity in the wholesale market, which reflects the true variable cost of generating electricity. This leads to inefficient energy use.
Policy Insight
If policymakers wish to improve efficiency in retail electricity markets, implementing a carefully designed critical peak pricing program and a simple time-of-use schedule can lead to meaningful improvements.
Introduction
Wholesale electricity markets—in which large customers like utilities buy power from generators —are both complex and diverse. In the broadest terms, generators submit bids containing quantity and price of electricity production. These bids can account for fuel costs, environmental taxes, and other operations and maintenance costs such as labor.
Independent system operators (ISOs) order all bids from highest to lowest to form the supply curve for electricity.1 They then intersect this supply curve with the total demand from all end consumers —residential, commercial and industrial—at each point in time. This process determines the wholesale market price, production level for each plant, and generation mix. And the process is repeated as frequently as every five minutes.
Prices fluctuate constantly as supply and demand conditions vary throughout the day. They can spike abruptly during periods of high demand or constrained supply. Prices also tend to be low during the night or when the weather is mild. There also exist forward markets for electricity, wherein traders attempt to predict the future price of electricity, based on historical electricity prices, predicted fluctuations in fuel prices, and weather forecasts. These markets typically act on day-ahead or hour-ahead timescales.
Responding to these rapidly changing prices would be incredibly difficult for the typical end user. However, most commercial and residential consumers do not directly interact with this market in their daily lives. They instead purchase their electricity from a local utility, which in turn has bought electricity on the wholesale market. Historically, these retail distributors have charged a single fixed-rate price of electricity.
This system creates a wedge between the wholesale and retail prices of electricity. That is, prices charged by utilities rarely match the current price of electricity in the wholesale market, which reflects the true variable cost of generating electricity. This leads to inefficient energy use— for example, during extreme peak hours when wholesale prices are unusually high, residential customers face a much lower price and do not have economic incentives to reduce their electricity use. To address this, utilities have been experimenting with various types of dynamic electricity pricing schemes, which allow the retail price of electricity to vary more frequently and to track the wholesale price more closely.
In a recent study, we (and our co-authors from MIT, UC Berkeley, and UC San Diego) use data over the period 2000 to 2020 for many of the largest wholesale power markets in the United States to analyze the performance of a wide range of hypothetical electricity pricing systems. We show that time-of-use (TOU) electricity pricing schemes are poorly correlated with real-time wholesale market prices and that this phenomenon is widespread across space and time (Hinchberger et al., 2024).
We consider two alternative pricing policies: time-of-use pricing, which sets a schedule of different hourly prices for different times of day, week, or year, and critical peak pricing in which a utility can “call” a limited number of peak events and charge a high price during hours when generation costs spike.
Trade-Offs in Retail Electricity Pricing
To provide customers with the right price signals and incentives to conserve energy, one may argue that retail prices should update in real-time with the wholesale price. This would resolve pricing inefficiencies but lead to an unpleasant and financially unsettling experience for customers.2 Wholesale electricity prices can be volatile, with negative prices in some hours3 and prices orders of magnitude greater than the annual average in others.
Allowing retail prices to vary with wholesale prices can lead to shockingly high electricity bills for some customers, especially when adverse weather events and other natural disasters cause more pronounced and frequent price spikes. Policymakers must balance these concerns with the benefits of providing consumers with adequate incentives to save energy during peak hours.
We compare candidate pricing schemes along three dimensions: 1) simplicity, 2) predictability, and 3) efficiency:
- Simplicity refers to the ease with which consumers can understand their electric bill. This can be roughly measured by the number of different prices consumers need to consider when planning their electricity usage over time—if they do this in the first place. Naturally, the standard single-price retail scheme is, as one might expect, the simplest. More complex pricing schemes complicate consumer decision-making. However, simpler tariffs are less able to capture variation in the wholesale market, causing the aforementioned pricing inefficiency.
- Predictability refers to the ability of retail customers to know the price of electricity in a given hour sufficiently in advance so that they can be incorporated into the decision process. With fixed prices, consumers are shielded from unpleasant “surprise bills”. However, wholesale electricity prices are difficult to predict far ahead of time, and therefore predictable pricing will be hard to align with market conditions.
- Efficiency, in an economic sense, refers to the case where customers pay the true variable cost of power, and therefore face the right incentives to conserve electricity when it is expensive. A fixed, single retail price causes over-use of electricity in peak periods and under-use in off-peak periods. Utilities and their customers could be made better off by shifting some consumption away from peak hours. This misallocation can also have environmental consequences, as it causes drastic shifts in load throughout the day. This means that electricity generation frequently occurs far along the merit order curve, using peak-load generation technologies that not only have higher variable costs but also often emit more pollution than base-load generators.
In the remainder of this policy brief, we consider the potential efficiency gains of alternative pricing schemes that sacrifice different amounts of simplicity and predictability. To analyze prospective policies, we have collected hour-level locational price data between 2000 and 2020 from wholesale markets across the United States, forming a dataset with over one billion observations. Our dataset covers all seven restructured independent U.S. system operators: PJM (East Coast), ISO-NE (New England), NYISO (New York), ERCOT (Texas) MISO (Midwest), SPP (South Central), and CAISO (California).
Sophisticated Time-of-Use Price Schedules
The first alternative policy we consider is sophisticated time-of-use pricing. These are pricing schemes that are fixed well in advance but can vary across different hours of the day, days of the week, or weeks of the year. An example from San Diego Gas & Electric is given in Figure 1, where prices vary by time of day and between weekdays and weekends.
The highest, on-peak prices apply to late afternoons and early evenings when power demand is high; the lowest “super off-peak” prices are charged at night and during other hours when power demand and wholesale-market prices are at their lowest.
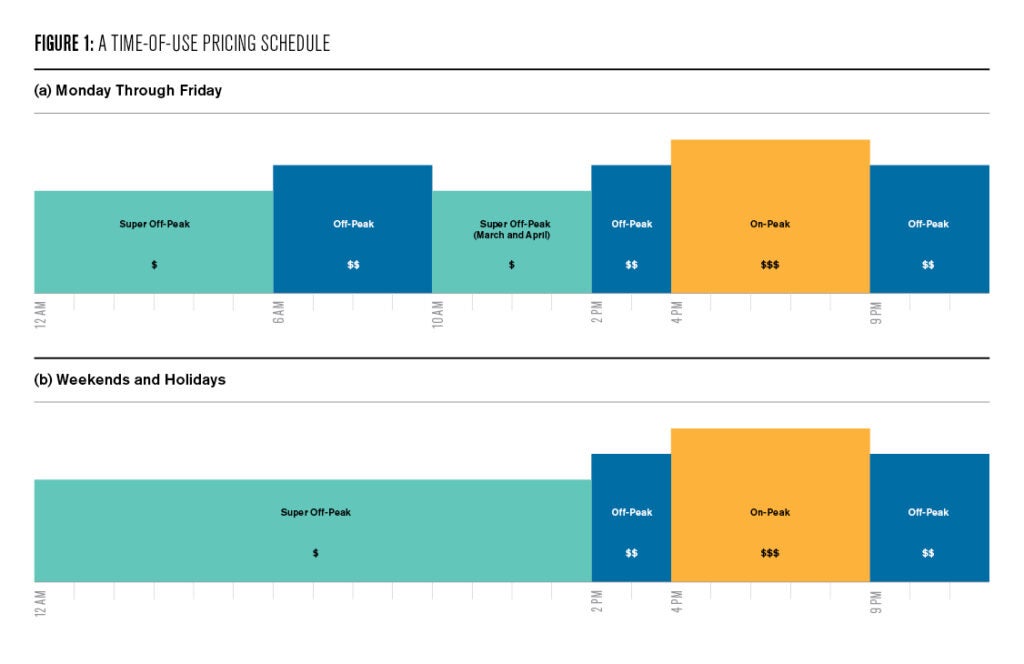
While the price of electricity will vary idiosyncratically hour by hour, utilities can still improve their tariff structures by taking advantage of cyclical patterns in prices. This variation can be thought of as occurring on three timescales: throughout the day, within the week, and across seasons. Within a day, usage (and, consequently, prices) are typically highest in the early evening and lowest in the middle of the night. Across days of the week, weekends (and holidays) experience smaller commercial and industrial loads compared to workdays. Over the course of the year, demand tends to be greatest during the summer months, when air conditioning units are most extensively used.
San Diego Gas & Electric includes all of these sources of variation in setting their price schedule, while other plans may include some. For example, Eversource in New England offers a monthly variable rate plan that only reflects seasonal variation, as well as a peak/off-peak pricing option that only reflects within-day variation. In general, a typical time-of-use schedule will feature peak hours during the early evening on weekdays, often “super-off peak” hours during the night, and some degree of seasonal updating.4
Using our data, we can construct hypothetical pricing schemes that take advantage of this cyclicality in different ways. Figure 2 displays the potential efficiency gains for four candidate pricing schemes: peak vs. off-peak pricing, peak pricing that can vary on weekdays vs. weekends, peak pricing by weekend/weekday and by season, and peak pricing by weekend/weekday by month-of-year.
The vertical axis represents how much (in proportional terms) the policy performs better than simply charging the average price, relative to the theoretical ideal of real-time pricing. In other words, 0 represents a policy that is as efficient as the best single-price policy, and 1 represents a policy that is as efficient as real-time pricing.
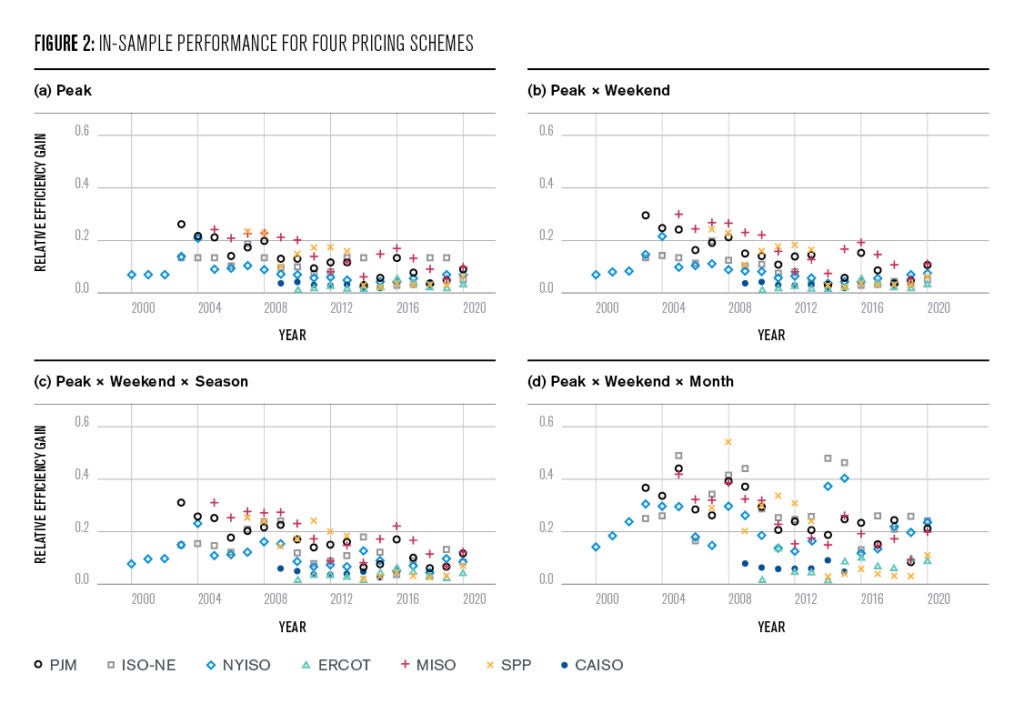
The result here is intuitive: with these hypothetical policies, greater complexity results in greater efficiency gains. Even though peak pricing appears to provide little benefit to the market relative to real-time pricing, these gains still amount to hundreds of millions of dollars per year on aggregate. However, these results here are not realistic for policy purposes. The price schedules in Figure 2 were generated “in-sample,” meaning that prices were set using data from the same year in which the prices were to be applied. Therefore, to obtain these results, price setters would need perfect knowledge of market conditions a full year in advance, which is simply not feasible.
The real task for a policymaker is to use previous years’ data to construct a price schedule that will most accurately predict prices in the coming year. In Figure 3, we model this, constructing the same four pricing policies as before, but instead using the pricing data from the three years before the policy is to be enacted. We then take the difference in efficiency between the candidate’s time-of-use policy and the policy that always charges the average price over the past three years.
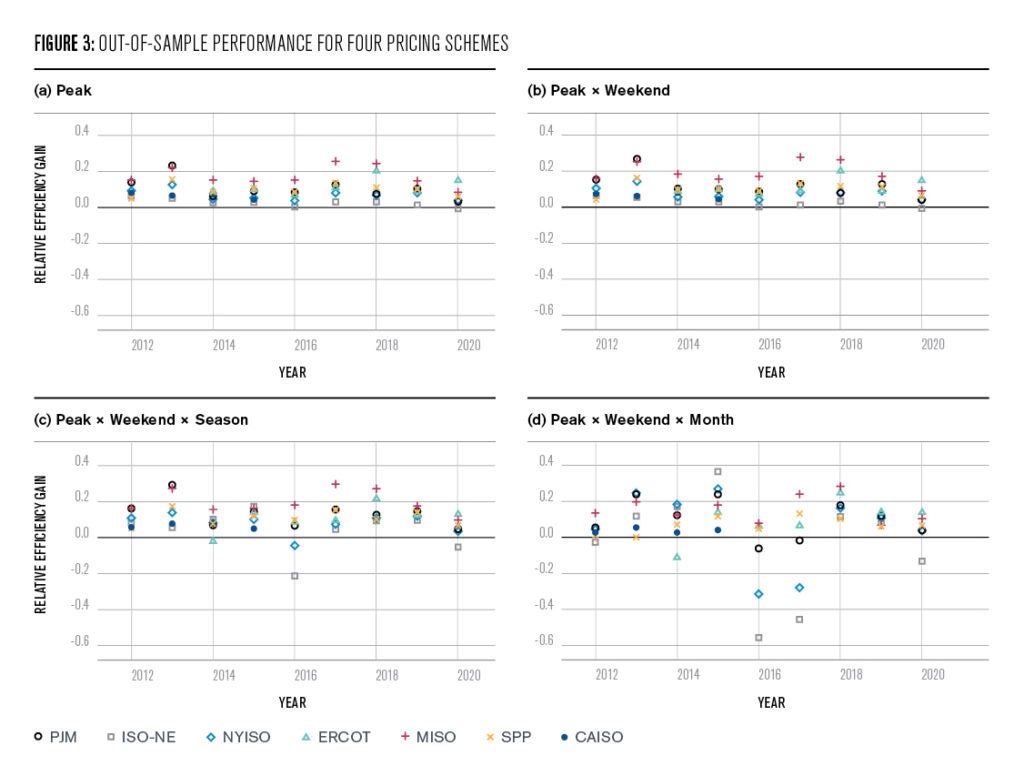
These results paint a less favorable picture of these policies—relative efficiency is improved on the order of 10 percent compared to a flat price. This still amounts to average annual efficiency gains of approximately $200 million across the seven markets. Strikingly, more complex policies do not render significantly higher average efficiency gains. The four panels of Figure 3 have mean efficiency gains of 9, 10, 11, and 7 percent, respectively. Additionally, more complex policies are more variable in their effectiveness—in fact, they often backfire and perform worse than a flat tariff, as shown by the negative values present.
In general, there are two sources of inefficiency in these schedules:
- There are forecasting errors in the average price: the average price of the policy that is set using data from the previous three years may differ substantially from the true average wholesale price in the year in which it is applied.
- There are imprecise targets of hourly prices: regardless of the average price, the “shape” of the proposed policy does not match the variations in the real-time market. For simple policies, this is largely driven by the lack of granularity in the schedule. For sophisticated policies, the level of granularity may seem to closely match the wholesale price, but because we are extrapolating from previous years’ idiosyncrasies, the shape that it forms is often incorrect. For example, the price of power on weekends in June at 5 p.m. may have been low in the past few years, but the current year might experience temperature spikes during those days and times.
Based on our modeling, setting electricity prices in advance using historical data has the potential to modestly improve efficiency. While simple policies offer gains on the order of 10%, sophisticated policies are not much better on average and often backfire. Complex time-of-use pricing is limited by the unpredictability of future real-time wholesale prices.
Critical Peak Pricing
The second alternative we consider is critical peak pricing. This describes a policy wherein utility companies can call some “critical peak events” in a calendar year.5 During these events, prices are either allowed to fluctuate freely with either the real-time/day-ahead power price or a predetermined “critical peak price.”
Typically, these events are restricted to peak daily usage hours and are called when prices are expected to be particularly high. This allows policymakers to mitigate the inefficiency incurred during the most extreme price events. The cost, however, is that consumers now must give up some of the predictability characteristic of the time-of-use schedules described above. They may experience cost shocks if they do not adjust their power usage accordingly during peak events.
We can again model this using our price data. First, we rank each day of the year according to the average day-ahead price during the peak usage hours of each ISO. We then construct price schedules that charge customers a fixed price except during these critical peak events, where that price is replaced by the day-ahead peak average.
Results averaged across all years of our data are shown in Figure 4, where the horizontal axis represents the number of critical peak events. The panel on the left uses event-level prices based on the day-ahead market, whereas the panel on the right uses a single price for all critical peak events.
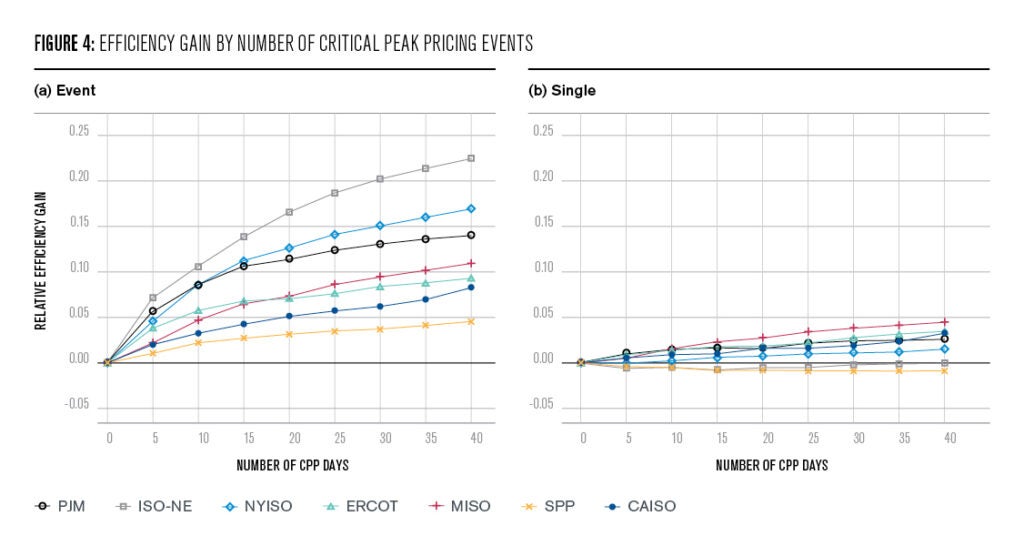
We see a striking difference between the two panels. With event-level pricing, we see efficiency gains of a similar magnitude to simple TOU policies using just twenty events. However, we see little overall benefit with a single critical peak price (CPP). The reason for this is straightforward: critical peak events are not all equally serious. A predicted wholesale price spike to $300/MWh is much more severe than a predicted price spike to $100/MWh. If utilities cannot signal this difference in severity between spikes, they are essentially left with a peak pricing scheme that only applies for a few days each year. An important benefit of a critical peak price is its ability to precisely incentivize conservation in crucial hours.
We also see significant differences in efficiency gains between markets. Why does ISO-New England (ISO-NE) benefit greatly from a critical peak price, while SPP doesn’t even break 5% after forty events? In part, this can be explained by differences in the predictive power of forward markets. Critical peak pricing depends on the ability of the day-ahead market to predict real-time prices to both call events optimally and price those events accurately.
Figure 5 shows the R2 (a measure of statistical fit) between hourly day-ahead and real-time prices for all seven markets. In the Northeast, forward and spot markets are strongly correlated throughout the years of our sample. In contrast, day-ahead markets in ERCOT, SPP, and CAISO, which have 20–30% of their generation supplied by wind and solar resources, are much worse predictors of real-time conditions. This makes critical peak price policies significantly less effective in these regions.
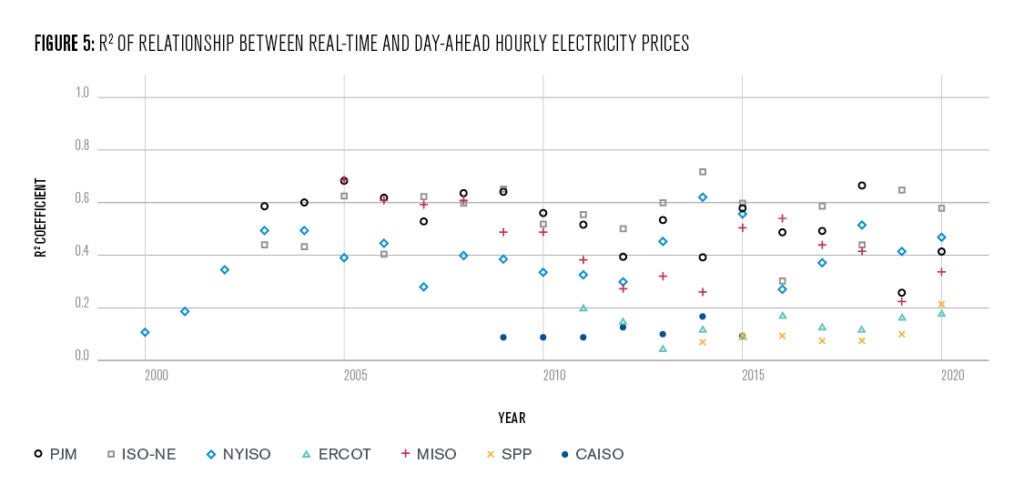
One might imagine that returns would be maximized if critical peak events were called very shortly before they occured using information from the real-time market. This would maximize the information used in calling these events. However, consumers would not have enough time to meaningfully react to these critical peak price levels, and the induced change in load would be insufficient to improve efficiency.
This approach raises concerns about the aforementioned “surprise bills,” unless regulators were to adopt a retail price cap (though this comes with its own set of inefficiencies). Empowering consumers to easily adjust electricity usage in response to sudden critical peak pricing events is paramount to improving market efficiency. Recent advances in home automation may offer avenues to capture some of the efficiency gains with less financial risk to the consumer.
Conclusion and Policy Recommendations
Both time-of-use and critical peak pricing have some potential to improve economic efficiency in retail electricity markets. However, these efficiency gains must be balanced against consumer concerns of simplicity and predictability. In designing time-of-use rates, we find that sacrificing simplicity does not lead to significantly better outcomes when price schedules are determined out-of-sample.
Simple peak-style schedules with between 2 and 8 price levels are sufficient to capture most of the potential welfare gains and are much easier for consumers to understand. In designing CPP rates, we find that sacrificing some predictability is necessary to earn robust efficiency returns. Specifically, we find that differentially pricing “very high” and “extremely high” load events is important – in practice, many utilities testing critical peak pricing policies are not doing so. We find that the benefits from these policies add up when used alongside each other: time-of-use targets routine cycles in prices, while critical peak pricing targets non-routine spikes.
If policymakers wish to improve efficiency in retail electricity markets, we recommend that they focus on implementing a carefully designed critical peak pricing program and a simple time-of-use schedule. This feasible policy has the potential to return between 15–20% of the welfare losses from imperfect pricing in the retail market without causing significant consumer confusion. As home automation advances in the years to come, and households can use software to shift power consumption away from high-price hours, policies could gradually shift toward real-time or day-ahead pricing. This would benefit consumers in the long run, as peak demand would go down, and less investment in generation capacity would be needed.
Arthur van Benthem
Associate Professor of Business Economics and Public PolicyArthur van Benthem is an expert in environmental and energy economics, exploring the economic efficiency of energy policy. He is a faculty fellow at the Kleinman Center and an associate professor of Business Economics and Public Policy at Wharton.
Andrew Hinchberger
Ph.D. Student, Northwestern UniversityAndrew Hinchberger is a Ph.D. student at Northwestern University studying energy and environmental economics and industrial organization.
Christopher Knittel
Director of the Center for Energy and Environmental Policy Research, MITChristopher Knittel is the George P. Shultz Professor and director of the Center for Energy and Environmental Policy Research at the Massachusetts Institute of Technology.
Mark Jacobsen
Professor, University of California San DiegoMark Jacobsen is a professor of economics at the University of California, San Diego and a research associate at the National Bureau of Economic Research. In 2015-2016, he was a visiting scholar at the Kleinman Center.
James Sallee
Professor, UC BerkeleyJames M. Sallee is a professor in the Department of Agricultural and Resource Economics at UC Berkeley, a research associate at the National Bureau of Economic Research, and a research associate at the Energy Institute at Haas.
Andersen, Laura M., Lars G. Hansen, Carsten L. Jensen, and Frank A. Wolak. 2017. “Using Real-Time Pricing and Information Provision to Shift Intra-Day Electricity Consumption: Evidence from Denmark.” URL http://web.stanford.edu/group/fwolak/cgi-bin/sites/default/files/into_versus_away_paper.pdf. Manuscript: Stanford University.
Borenstein, Severin. “Customer Risk from Real-Time Retail Electricity Pricing: Bill Volatility and Hedgability.” The Energy Journal 28, no. 2 (2007). https://doi.org/10.3386/w12524.
———. 2005a. “The Long-Run Efficiency of Real-Time Electricity Pricing.” The Energy
Journal 26 (3):93–116.
———. 2005b. “Time-Varying Retail Electricity Prices: Theory and Practice.” In: Electricity
Deregulation: Choices and Challenges, edited by Steven Puller and James Griffin. University of Chicago Press: Chicago, IL, 317–357.
Borenstein, Severin and Stephen P. Holland. 2005. “On the Efficiency of Competitive Electricity
Markets with Time-Invariant Retail Prices.” RAND Journal of Economics 36 (3):469–493.
“Business Practice Manuals for Market Instruments Version 73.” California ISO, August 25, 2022.
Clark, Erin. “His Lights Stayed on During Texas’ Storm. Now He Owes $16,752.” The New York Times, February 21, 2021. https://www.nytimes.com/2021/02/20/us/texas-storm-electric-bills.html.
Commonwealth Edison. “Hourly Pricing.” Accessed June 3, 2024. https://www.comed.com/WaysToSave/ForYourHome/Pages/HourlyPricing.aspx.
Commonwealth Edison. “Peak Time Savings.” Accessed June 3, 2024. https://www.comed.com/WaysToSave/ForYourHome/Pages/PeakTimeSavings.aspx.
“Critical Peak Pricing.” San Diego Gas & Electric. Accessed June 3, 2024. https://www.sdge.com/businesses/savings-center/energy-management-programs/demand-response/critical-peak-pricing.
Eversource. “Electric Supply Rates.” Accessed June 3, 2024. https://www.eversource.com/content/ema-c/residential/account-billing/manage-bill/about-your-bill/rates-tariffs/electric-supply-rates.
Eversource. “Variable Peak Pricing (VPP).” Accessed June 3, 2024. https://www.eversource.com/clp/vpp/vpp.aspx.
Fabra, Natalia, David Rapson, Mar Reguant, and Jingyuan Wang, 2021. “Estimating the Elasticity to Real-Time Pricing: Evidence from the Spanish Electricity Market.” AEA Papers and Proceedings, American Economic Association, vol. 111, pages 425-429, May.
Gillan, James. 2017. “Dynamic Pricing, Attention, and Automation: Evidence from a Field Experiment in Electricity Consumption.” Accessed June 3, 2024. http://jamesgillan.info/s/JMP_Gillan_most_recent_draft.pdf. Manuscript: UC Berkeley.
Hinchberger, Andrew J., Jacobsen, Mark R., Christopher R. Knittel, James M. Sallee, and Arthur A. van Benthem. “The Efficiency of Dynamic Electricity Pricing Schemes.” (working paper, 2024).
Ito, Koichiro, Takanori Ida, and Makoto Tanaka. 2018. “Moral Suasion and Economic Incentives: Field Experimental Evidence from Energy Demand.” American Economic Journal: Economic Policy 10 (1):1–29.
Jacobsen, Mark R., Christopher R. Knittel, James M. Sallee, and Arthur A. van Benthem. “The Use of Regression Statistics to Analyze Imperfect Pricing Policies.” Journal of Political Economy 128, no. 5 (2020): 1826–76. https://doi.org/10.1086/705553.
Jessoe, Katrina and David Rapson. 2014. “Knowledge is (Less) Power: Experimental Evidence from Residential Electricity Use.” American Economic Review 104 (4):1417–1438.
Kato, Takaaki, Ayano Tokuhara, Yoshiaki Ushifusa, Arata Sakurai, Keiji Aramaki, and Fumitaka Maruyama. “Consumer Responses to Critical Peak Pricing: Impacts of Maximum Electricity-Saving Behavior.” The Electricity Journal 29, no. 2 (2016): 12–19. https://doi.org/10.1016/j.tej.2016.02.002.
PECO. “Time-of-Use Pricing.” Accessed June 3, 2024. https://www.peco.com/SmartEnergy/InnovationTechnology/Pages/TimeOfUsePricing.aspx.
San Diego Gas & Electric. “Energy Plans for Every Lifestyle.” Accessed June 3, 2024. https://www.sdge.com/residential/pricing-plans/about-our-pricing-plans/whenmatters.
Wolak, Frank. “Residential Customer Response to Real-Time Pricing: The Anaheim Critical-Peak Pricing Experiment.” Center for the Study of Energy Markets (CSEM) Working Paper Series 2006.
- This supply curve is known as the “merit order.” [↩]
- Nevertheless, some utilities are experimenting with these sorts of policies, such as ComEd’s opt-in Hourly Pricing program. [↩]
- Some power generators would rather pay to stay online for a few hours in order to avoid costly shutdown or to monetize a production tax credit. [↩]
- The authors’ local utility, PECO, has a time-of-use pricing scheme that is a good example of this. [↩]
- This number can vary: consider ComEd’s “1 to 2 days” to SDGE’s “up to 18 days.” [↩]