Artificial Intelligence for Smart Buildings
Managing energy demand is essential in the complex global energy transition where price volatility is the norm, and Rahul Mangharam is making demand-side energy flexibility management a more powerful tool in energy policy.
At a time when climate change is producing extreme swings in temperature, energy prices themselves have grown wildly volatile. Professor Rahul Mangharam has seen instances in the summer when energy prices went from $32/MWh to $800/ MWh—a 36x increase in price—in a matter of five minutes. In the winter during polar vortexes, the price can jump from $25/ MWh to $2,680/MWh, and the grid requires the demand-side to become flexible.
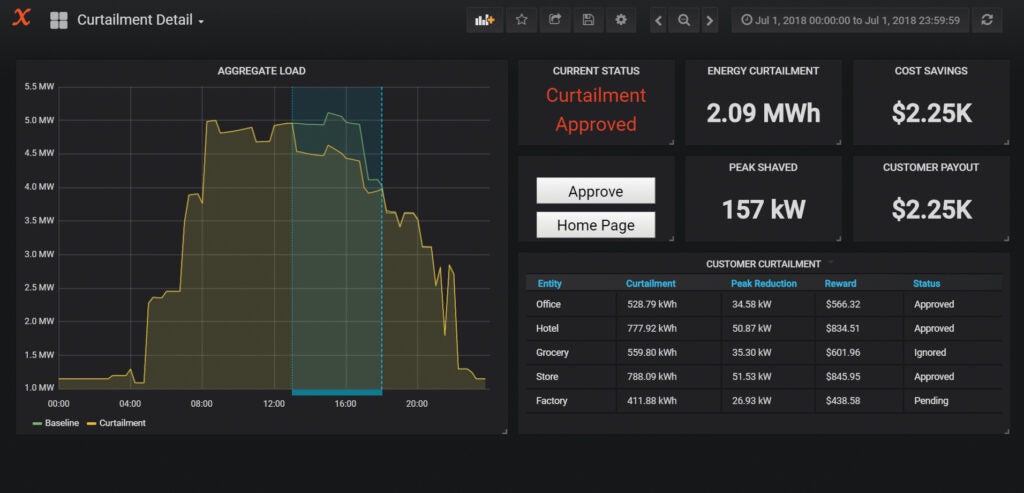
Mangharam is interested in buildings because they consume the bulk of energy in the grid. And 70 percent of electricity is consumed by heating and cooling systems. “Our goal is to take buildings from not-so-smart on/off rule-based systems to electricity price-aware and adaptive smart buildings,” Mangharam says.
For Mangharam, the big question is: “How can we transform buildings into aggregate flexible loads that adapt their usage to spikes in prices? As buildings are unique and complex, we bridge machine learning and control theory for low-cost and low-touch methods of controlling and aggregating building loads.”
He’s not just interested in making building operations more cost-effective, but in the climate consequences of buildings that can’t adapt better to extreme weather. When there is a price spike, it’s due to a grid operator turning on its peak-capacity systems—often these are old, coal- burning plants.
“How can these buildings become responsive to this volatility?” Mangharam asks. The core of his research uses machine learning algorithms to capture a building’s dynamics through hundreds of sensors already in the building. In a few hours, a data-driven model of the building is learned to predict future power consumption. This model is used to generate automatic control strategies to reduce the building’s energy consumption, while guaranteeing thermal comfort.
We are bridging machine learning and control systems for the demand-side of energy. Our algorithms continually evaluate how much energy flexibility a building has and automatically controls it for sustainable operations with budget certainty. From a budget standpoint, there’s lower cost and more predictability. For facilities, less guesswork and more visibility. For occupants, more comfort. And for sustainability, there’s a smaller carbon footprint,” he says. This way, as the prices of energy go up, the building management system can respond in real time.
For cities, universities, or other major property owners, these price spikes are extremely expensive. Penn, for example, has 217 buildings that altogether cost $28 million per year for electricity usage. In years past, if Penn went over capacity for, say, 30 minutes, the demand charge would be $720,000—and, the university’s electric bill would be higher going forward to account for this peak usage. How smart is that?
Policy Challenge
Public Utility Commissions in more than 30 states approved Demand Response (DR) programs, and utilities have enrolled at least 100MW of DR capacity. PJM, which manages the transmission market in 13 eastern and midwestern states, met over 4 percent of its 2018 committed capacity using DR. The interactive analytics dashboard of Mangharam’s system, which micro-grid operators use to compare real- time energy flexibility across their portfolio of buildings.
The Numbers
According to the International Energy Association, the buildings and building construction sectors combined are responsible for 36 percent of global final energy consumption and nearly 40 percent of total direct and indirect CO2 emissions.
Further Reading
Our goal is to take buildings from not so smart on/off rule-based systems to electricity price-aware and adaptive smart buildings.
Rahul Mangharam