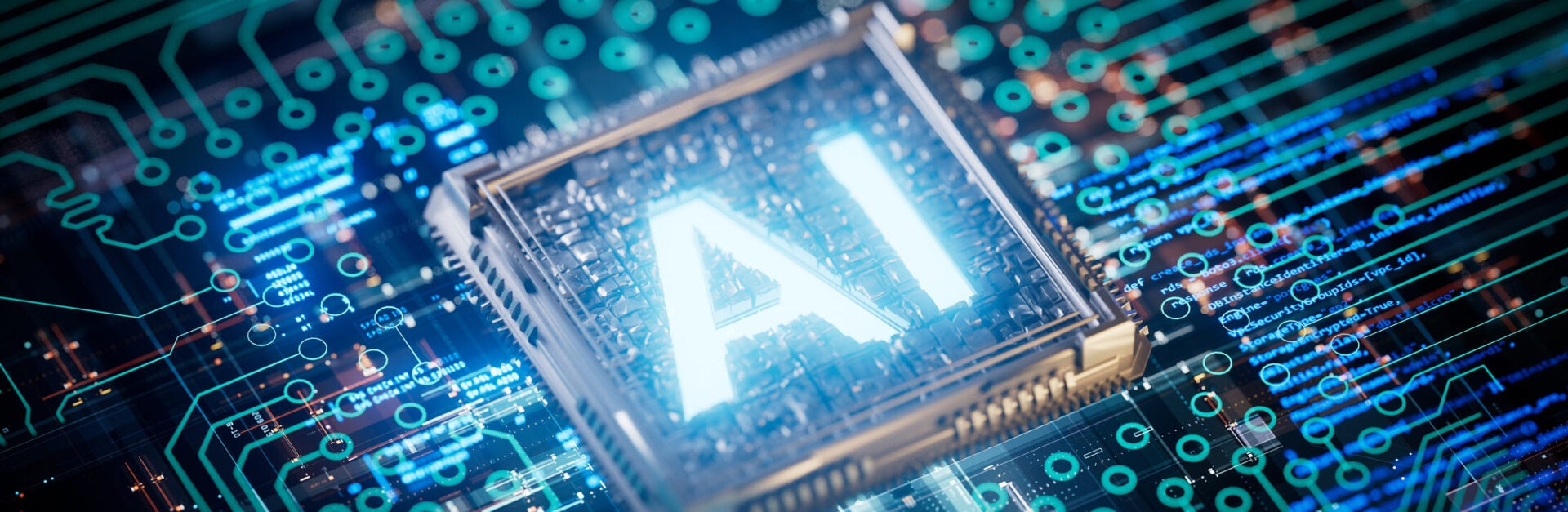
Why AI Consumes So Much Energy and What Might Be Done About It
Nvidia’s director of accelerated computing, and a Penn expert in AI and datacenters, explain why AI uses so much energy, and how its energy appetite might be curbed.
Artificial Intelligence is taking off. In just under two years since the introduction of Chat GPT, the first popular AI chatbot, the global number of AI bot users has grown to one and a half billion. Yet, for the U.S. electricity grid, AI’s dramatic growth could not have come at a more challenging time. AI is energy-intensive, and its expansion is putting additional strain on an already burdened grid that’s struggling to keep pace with rising electricity demand in many regions. In addition, AI’s energy demands complicate efforts to decarbonize the grid as more electricity–generated with a mixture of carbon-free and fossil fuels–is required to support its growth.
The podcast explores the challenges AI presents to the power grid with Dion Harris, Director of Accelerated Computing at Nvidia, and Benjamin Lee, a professor of electrical engineering and computer science at the University of Pennsylvania. The two explain how and why AI leads to increased electricity use and explore strategies to limit AI’s energy impact.
Andy Stone: Welcome to the Energy Policy Now podcast from the Kleinman Center for Energy Policy at the University of Pennsylvania. I’m Andy Stone.
Artificial intelligence is simply taking off. In just under two years since the introduction of ChatGPT, the first popular AI chatbot, the global number of AI bot users has grown to more than one-and-a-half billion. Yet for the US electricity grid, AI’s dramatic growth could not have come at a more challenging time. AI is energy-intensive, and its expansion is putting additional strain on an already burdened grid that’s struggling to keep pace with rising electricity demand. In addition, AI’s energy demands complicate efforts to decarbonize the grid, as more electricity generated with a mixture of carbon-free and fossil fuels is required to support AI’s growth.
On today’s podcast, we’ll explore the challenges AI presents to the power grid with two guests. Dion Harris is Director of Accelerated Computing with Nvidia, the company that supplies the majority of the world’s AI computing chips. Benjamin Lee is a Professor of Electrical Engineering and Computer Science at the University of Pennsylvania and a Visiting Researcher at Google’s Global Infrastructure Group. The two will explain how and why AI leads to increased electricity use, and they will explore strategies to limit AI’s energy impact. Dion and Ben, welcome to the podcast.
Dion Harris: Thanks for having us.
Benjamin Lee: It’s wonderful to be here.
Stone: So Dion, your role with Nvidia has you deeply involved with AI data centers and data center growth. Could you tell us about your role and the intersection with the energy challenges that we’ll be discussing today?
Harris: As you mentioned, as Director of Accelerated Computing, Nvidia has long been a champion of an alternative approach to computing, which has traditionally been general purpose CPU-based computing. We started pioneering accelerated computing, looking at every domain to try to deliver more performance and efficiency. This is really where we’ve positioned ourselves within the data center. With the advent of AI, being a killer use case for accelerated computing, we’re now looking at how we can stretch the bounds of what’s possible in terms of AI capabilities while also growing as efficiently as possible.
A lot of what we’re doing with Nvidia is looking at the entire data center. How can we optimize our platform at the compute, at the networking, at the software layer in order to help our customers and partners deliver the performance they require with the lowest power envelope possible? So that is, in essence, what we spend most of our time doing at Nvidia, really making sure that we can get the most out of the lowest possible resource requirement for our customers and partners.
Stone: And Ben, you’re a Professor of Engineering here at Penn. Introduce us to your work on data centers and AI.
Lee: I’m a computer architect by training, which is to say that I think a lot about how I’m to design microprocessors, memory systems, and the data centers that house them. We’ve increasingly been interested in the data center context because the power numbers and the energy efficiency needs are just really so great. Over the past three to five years, we’ve been looking at path-finding and figuring out what the solution space looks like for next generation data centers. This requires rethinking how we provision power, how we design hardware for those data centers, and then how we manage those data centers to use power more efficiently and manage the software workloads within them. This is a much larger effort, actually. We have just recently been awarded a fairly large program from the National Science Foundation involving 14 other professors to really take a top-to-bottom look at this question ranging from semiconductor manufacturing all the way up to data center operations. I think we’re really just getting started. We have a sense of the problem, and we have a sense of the solutions, but we really need to better understand the numbers and optimize the solution.
Stone: We’ve established generally that AI is very energy intensive. Dion, I want to ask you if you could frame the magnitude of growth in AI and AI-driven energy consumption, particularly over these past two years, what trajectory are we on in terms of percentages of growth looking forward?
Harris: Yes, if I just describe the overall compute that is required, when you look at how the changes have happened in the data center as it relates to AI, it was really around 2018, 2019 where you really saw AI take off. If we go back a little bit further, machine learning has been around for decades, but it really wasn’t until we had that sentinel moment where we had AlexNet demonstrate some super communicator capabilities on image classification capabilities, and we saw a huge interest in leveraging accelerated computing to go and tackle some of the AI problems.
But as we got to around 2017, there was a paper by Google that was about the transformer, and that was really what, in my mind, really kicked off a huge interest in understanding how we could explore the possibilities and capabilities of AI by leveraging more compute. Now, like I mentioned, when you think about that trajectory from 2018 or so to 2023, we saw a huge influx of the number of models. We saw a huge influx in the actual compute required to go in and support those models or training workloads. But again, as I mentioned at the onset, Nvidia has been rapidly evolving our platform to try to get ahead of that demand. So when you think about the figures that are out there, most people describe the data center as consuming anywhere from 1 to 2% of electricity that’s consumed globally. And of that, when you think about AI, they say roughly about 12% of that data center footprint today is occupied by AI-based workloads.
So in this context, today the current footprint of AI is roughly about .1 to .2%, depending on the numbers you’re looking at. But I think the thoughts or interest is understanding how we continue to keep up with the demand for what’s happening in a lot of these AI development spaces — not just training, but also for inference — and how we make sure that we can not have the growth outpace our ability to offset the overall impact on the environment and the grid. And so in terms of those numbers, we’re doing lots of different projects to help offset that and hopefully curb that growth trajectory.
Stone: You said just a moment ago that about 12% of data center energy use is dedicated to AI purposes. It’s interesting because, from my understanding, the standard chatbot query that you would use in AI chatbot — that’s obviously many more uses — but on that level, that is about ten times the level of energy intensity or energy use as a standard Google search. Is that correct?
Harris: There have been some numbers that have described that dynamic, and you’re right. There are a couple of different researchers that have mapped that out. And I think there is also research that is really trying to understand the longitudinal effect of AI in being deployed. For example, when you do that one query, are you done? Or are you going and clicking on several other links to understand how and where that information that you’re trying to pull together is?
So I think those studies that are really trying to understand the impacts of AI versus traditional computing are also being fleshed out, but then that’s also compounded with the fact that now AI is being included in general work research, as well. So to your point, I think right now as a society, we’re really excited about how and where we can leverage AI, and being able to understand where the benefit outweighs the potential costs is really where we are. I think a lot of the data points that are starting to come in are giving us some texture around that, as well.
Stone: I want to ask you one more question on this, and I don’t want to get too deep into the weeds of the technology here, but I think it’s just an interesting point. From my understanding, AI and the processes are relying upon graphics processing units, GPUs. These are different from the CPUs that are used generally in data centers, I guess, up until this point. Tell us a little bit more about that and how that relates to energy consumption.
Harris: That’s a great question. When you think about what accelerated computing is — and again, I started the call describing our role in spearheading this whole new computing model. What it means is you’re taking a GPU, in this case, an accelerator being a GPU, and you’re including that in a server that already has a CPU. So you ask, “Why would you do that?” because you’re adding power load, and you’re also adding cost to that server.
The reason why you would do that is because it can actually create more compute density and ultimately more overall efficiency. One analogy I like to use is thinking about our transportation system. When you think about how we look at energy efficiency on the roads, we say, “Okay, you have people who drive their personal cars, and you also have public transportation.” At a nominal level, you would say your car consumes a lot less gas or fuel or energy than a diesel bus, but when you look at the number of bodies and the work that can get moved around in that diesel bus, it’s a much more efficient way of getting that work done, getting people to and from work, as an example.
So in essence, adding a GPU to those workloads can typically translate into orders of magnitude, or at least several x-factors overall improvement in the productivity of that asset, meaning the amount of work you could get done for the same amount of power, for the same amount of money, or for the same amount of floor space. And so that in essence has been why accelerated computing has been really powering this whole sort of transformational shift, not just in AI, but in computational fluid dynamics, in climate and weather, in material science, in you name it — data processing. And so this whole revolution, if you will, around AI has really been the tip of the spear of the bright, shiny object, but accelerated computing has been touching a number of different workloads, leveraging that GPU that you asked about.
Stone: So AI presents a major challenge for the electricity system, and I think these challenges might be broadly viewed as being one of two types. The first is that, particularly in certain areas of the country, AI-driven energy growth is outpacing the speed at which new sources of energy supply can be added to the grid. This comes as some of the largest electricity markets have already indicated concern about the ability of the grid to reliably meet demand going forward.
The second concern revolves around the climate impact of AI, and we’re already seeing some of the major technology companies, the companies that operate data centers, having to push out their decarbonization targets because AI consumes so much energy. So Ben, to get us started on this one, to what extent does the interpretation of AI energy challenges that I’ve just kind of laid out here — to what extent does it match the view that you see of the challenges before us?
Lee: I would agree that, first and foremost, there has been a lot of data center construction over the past few years, since the advent of generative AI. And I think that’s mainly because many of these big technology companies are still exploring, figuring out where those benefits are greatest, and as a result, there is a lot of anticipation or a lot of speculative roll-out of infrastructure to prepare for the inference side. I think the training side — yes, we want to train new, increasingly capable models, and I think there’s a lot of fundamental research in figuring out how to extend these models beyond language, to other modalities like video and audio and other formats. I think there has also been a lot of interest in taking the models we already know how to train and models that have performed well and finding those use cases, those applications. That’s where a lot of the uncertainty is, because we don’t know the extent to which users will adopt AI in their everyday life. We see, as Dion mentioned earlier, AI getting integrated into search queries, right? So a lot of AI has been integrated behind the scenes and also increasing the computational costs of office productivity and search engines. So I think that’s really driving a lot of the growth and demand for electricity, and as you mentioned, data center construction is unevenly distributed throughout the United States. I think Northern Virginia is often mentioned as a place where a lot of data center construction is happening. And then there’s the concern about whether or not that will affect that particular region’s ability to reduce their carbon footprint.
So I would say that that’s the first and foremost concern that folks have about data center compute. Secondarily, I would say that there are interesting questions about supply chain issues, where we don’t have a lot of good data, the extent to which we are emitting embodied carbon from the manufacture of semiconductors. And we’re manufacturing quite a bit more than we used to, most of it in East Asia.
So I think that there’s an understanding in trying to quantify many of these issues. I would say that the electricity piece, we have a rough sense of how to address because more renewable energy, maybe utility-scale batteries, maybe more investment in efficient data centers. All of that will help with the energy side of it. But then there’s also the manufacturing side that needs to be considered when we think about carbon holistically.
Stone: Dion, to what extent do you see the energy problem as a potential headwind to AI growth?
Harris: It’s interesting because you look at where we are today, and like you said, there is kind of a short-term and near-term perspective, right? When you think about the short term, in terms of getting more day extension online, getting more power and tapping into grids. Obviously there are lots of long delays, and most of our customers are seeing and experiencing that. A lot of what they’re trying to figure out is, “How can I get the most done with the infrastructure I have or the power envelope I have for this given data center or given facility?” And so that’s where, like I mentioned before, there’s a huge focus on figuring out how and where they can leverage accelerated computing first of all, but secondly, how and where they can leverage using the most advanced architecture, if you will, to drive the most efficiency. And so because a lot of the improvements that we’re making generation over generation are typically x-factors of improvements, you’ll see that there’s a strong desire and emphasis to move to that latest gen platform in order to drive more efficiency and to make the most out of their limited power availability.
So, do I think there’s a limit in terms of AI moving forward, given some of these constraints you highlighted? I don’t think so. I think there’s certainly a challenge because it’s not just data centers. It’s going to involve working with the grids. It’s going to involve working with policy-makers, et cetera, to meet a lot of those challenges. But I think, based on what we’ve seen so far, it seems like there’s lots of opportunity and momentum in that way. When you think about what happened in the 2010 to 2019 timeframe, where there’s a huge emphasis and focus on driving the overall efficiency of the data center through PUE metrics, you saw a huge downshift there. I think there’s going to be a similar transition, where you’re looking at how you drive the efficiency of the overall infrastructure, not just in terms of PUE, in terms of what power is getting utilized, but the actual work that’s getting done, and I think that transition is happening right now, as I described going to accelerated computing and leveraging it for AI and other workloads.
So in short, I think there are challenges for sure, but it feels like where the market is today and where a lot of the innovation is happening, I think there shouldn’t be a negative impact on overall AI growth because there are all these exciting innovations happening.
Stone: That’s an interesting point. Would it be fair to say that we’re still relatively in, I guess, the very early days of AI, and that there’s a lot of work to be done and a lot of opportunity in terms of the efficiencies of the technology? Any sense — and this may be an impossible question to answer — but is there any sense of how much headroom there is, in terms of future efficiency improvements? Or is that just really TBD?
Harris: It’s kind of TBD, but some of the promising signs, I’ll just point to all the different innovations that are happening. I mentioned accelerated computing, so I won’t talk about that as much, but everything is happening in the compute for sure to drive more efficiency. But there’s a lot of work that’s being done in the networking and how the systems and GPUs are connected, meaning how can you drive more density, more efficiency through having all these processors more tightly coupled? And that is inherently increasing the efficiency of the compute stack.
And then there’s all the software and algorithmic work that’s being done, whether it’s improving the models themselves, during training and inference. The media just announced something called the “Nvidia Quasar Quantization Platform,” which is really about how can we improve the algorithmic efficiency in leveraging lower precisions? And making sure that during inference, when models are deployed, you’re able to service those models in the most efficient possible way across the infrastructure.
So all of these things are happening at the same time, and then the innovation that’s happening in terms of the models themselves, like you said, we’re very much in a nascent state of AI right now. And as we’re embarking on the next wave of AI, in terms of it being deployed and used, you’re going to see even more innovations that are being used to help deploy these models more efficiently and use them to ultimately drive productivity and efficiency. I think that’s the other thing I’ll just close on, is saying I think that there is a longitudinal effect of AI that is also still trying to be understood. In other words, when you’re using AI, for example, in CFD-type work — computational flow dynamics. Today that consumes over 20 billion CPU core hours. If you’re able to offset that using AI approaches that can be done in a fraction of the time and energy, there are some savings there that we think, as these models get deployed and used more broadly, that will start to accumulate as well.
So the fact that we’re in the early stages of the model development, we’re also in the early stages of the model deployment and truly understanding where there are both pros and cons, in terms of how it will affect overall energy consumption.
Stone: Ben, you recently co-authored a paper that describes two sources of greenhouse emissions from AI. One is “operational emissions,” and the second is what’s known as “embodied emissions.” I wonder if you could begin with the first, these operational emissions? Explain to us what they are and how significant they are.
Lee: Right, I think the operational emissions are primarily associated with electricity use. There has been a lot of effort in reducing the carbon footprint of the energy going into data centers. Much of this has actually been driven by the technology companies themselves. They are investing huge amounts into wind and solar installations. As a result, they are getting these power purchase agreements in place, and then also getting credits for renewable energy being generated.
Now many of these technology companies will say that at the end of the year, when they compare the credits that they have received against the energy consumed in their data centers, if those numbers line up — and that’s the basis for their net zero claims today. So operational carbon, again, is the issue around how energy is generated, to what extent that energy is carbon-intensive, and to what extent that energy is going to data center computing. I would say that there is a little bit more that could be done, a lot more that could be done in this space because ultimately we are talking about offsets, where the renewable energy that’s being generated doesn’t necessarily line up with hours in the day where a lot of the compute is happening. So to the extent that we could store some of that carbon-free energy when it’s available on the grid abundantly, or to the extent that we could reschedule some of the computation so that we compute more when there’s more sunshine or more wind and compute less, otherwise. I think that would make a big difference in reducing the operational carbon.
Stone: I think also, and I think Dion started to talk about this as well, there are also the issues of consumption, as determined by how much energy model development and model training takes. I think there might be, to some extent, an opportunity to do less remodeling, for example, when ChatGPT 3.5 goes to 4 or 4.5. And I’m no expert on this, but I think there’s some degree of remodeling that goes on there that is very energy intensive. Maybe some of that can be taken out in the future, as well?
Harris: I’ll just jump in here and say some of what we’re also seeing is some of the placement of those workloads are starting to be impacted or being at least thought through a little bit differently. In other words, you mentioned training. A lot of those training workloads, where they don’t necessarily need to be in proximity to users in densely populated areas, can be placed where renewable resources are more abundant and available. And so that is starting to happen as well, where you see a lot of the training data centers taking hold in less densely populated areas that have lower impacts on some of those environments.
And another thing, like you said, where you talk about the inferencing capabilities, in terms of how those are being deployed. Again, lots of smaller-edged workloads that can still satisfy the need and the latency requirements of users but can be done in a much more efficient way. So I think there is now a much more thoughtful approach to, where you mentioned before. Before, it was just the high concentration of data centers in a given area. Now there’s, I would imagine, a much more thoughtful approach where we’re seeing a better distribution of those AI training data centers in particular, where they’re being located and how they’re being leveraged to consume less resources.
Lee: I would agree with that, and I would also say that as these models become more mature, especially on the language side, there may be an opportunity to train a little bit less and maybe just refine them incrementally as more data or particularly, use cases, arise. That would dramatically reduce the footprint of training costs. Of course if you start talking about other modalities like images or video, that may increase training costs with different classes of models.
I think there are a lot of really interesting directions and research areas that are trying to reduce the cost of training. Likewise, for inference, Dion mentioned this a little bit earlier, the notion of compressing the models, quantizing, shrinking the models to the extent possible. There is a huge amount of research among academics on this, as well as in the industry, and I think we’ll see more and more of these techniques come online, and that will also improve the efficiency. So I think the relative mix of energy spent on training versus inference is not quite clear. We think that both are fairly significant at this time, though.
Harris: Yes, and another technique that is being leveraged is something called “retrieval-augmented generation” or RAG. It speaks to your point around not needing to retrain a model every time you want to create a very targeted use case. So RAGs are basically leveraging vector data bases that allow users to basically incorporate their proprietary data or their specific terminology or use case specific content within that foundational model. So it gives you more specific model capability, without having to go and retrain a new foundational model on that use case.
So those are some examples. We mentioned fine-tuning and other elements of the model development process that are actually happening to help make sure that that’s requiring less energy and being more efficient.
Stone: Ben, in your research, you also go into what’s called “embodied carbon,” and I believe this has something to do with the hardware that’s involved in developing data centers. One of the key issues here, as AI grows rapidly in data centers generally, that implies a lot of new physical infrastructure that also carries its own carbon content. Can you tell us a little bit more about this embodied carbon and some of the understanding at this point on how that may be minimized?
Lee: Right, so the embodied carbon really relates to the manufacture of hardware components that go into servers. So we’re talking about the processors, the memories, the storage systems, and so on. One easy way to think about it is that the embodied carbon footprint roughly increases proportionally with the amount of silicon area that you are fabricating. So a larger chip will tend to have more carbon emitted during its manufacturing process.
For example, one of the largest sources of embodied carbon within data centers would be in the memory systems, because there are just so many memory chips sitting in these servers, and we’re talking about terabytes per server and hundreds of thousands, potentially, of these servers. So accounting for the cost of manufacturing these chips is a fairly significant effort, partly because it requires understanding data from so many of the supply chain entities and individuals or companies that contribute. So for example, you might be collecting data from Intel and Nvidia from their processors. You may go to Samsung and Hynix for understanding about the memories, and other partners for solid-state disks. And because their accounting methodologies are still rather immature, it’s hard to know under what conditions you’re comparing apples and oranges.
So I think that’s a grand challenge, as many in the industry are thinking about now, how to harmonize the accounting and get a better sense of the numbers. In terms of mitigating the sources of embodied carbon, half of the embodied carbon is coming from energy use at the fabs, so we’re talking about these fabrication plants in Taiwan or Korea. Half of the embodied carbon is associated with the gases and the chemicals that are being used to etch the semiconductors, the lithographic processes.
The energy use, we could probably mitigate with energy storage, with renewable energy investments. East Asia isn’t particularly great at the moment about adopting renewable energy, but we can think about strategies to improve those numbers. But those chemicals and those gases associated with fabrication tend to be harder to abate. I think one of the questions we have in data center construction is the extent to which we can reduce, reuse, and recycle the hardware components — maybe selectively refresh different types of hardware at different rates, based on the technology cadence, or thinking a little bit harder about how much more capability we’ll get from that next generation of hardware. I think thinking a little bit about procurement is going to be important.
Stone: I want to stay with that point for just a moment because looking at the last 20 years, when we look at the development of the computer systems that we have today, there have been so many generations of new hardware that have come out. I’m thinking in general computing terms, but new software needs more powerful hardware, and that implies many generations of this hardware, which can be carbon-intensive, being replaced. Is there a way — and I think you just started to talk about it — that there can really be a focus on making sure that the new AI technologies are capable of running on existing hardware for longer?
Lee: I think in the data center context — I’ll go back to something that Dion said, which is to say that GPUs are much more energy efficient than CPUs for the classes of workloads we’ve been talking about. Their energy efficiency may be improving at a faster cadence because of the renewed interest in these platforms. So when you think about replacing hardware, the question is: I’m going to get some performance or energy efficiency advantage by buying a new chip. Is that advantage large enough to justify the capital cost or the manufacturing cost associated with that chip? I think for some hardware components at the bleeding edge, that answer may very well be yes. You’re going to get better energy efficiency with new hardware. But in other cases, the answer might be no.
I’d also say for consumer electronics, and this is where the costs are most obvious — I think you’ll see, for example, in your phones a very high refresh rate. I guess it’s slowing down a little bit nowadays, but because there’s so much silicon in your phones, and because your phones draw relatively little power, I think that’s where we’re probably most concerned about embodied carbon costs — consumer electronics, VR headsets, phones, tablets, laptops, et cetera.
Stone: Ben, there’s one other concept that you bring up in the research. This is the concept of energy proportionality. The way I understand it is energy consumption would rise and fall with computing demand, which I guess is somewhat novel. Could you explain more about that?
Lee: Right, so the idea behind energy proportionality really is, as you say, as utilization of your chip increases, so does its power profile. Conversely, as you compute less on that chip, the power profile of that chip should go down. Classically, in central processing units and CPUs, you typically see this implemented with some sort of frequency scaling. The processor or the chip runs faster when there’s more work to be done and runs slower when there’s less work to be done.
I think that sort of dynamic power range is going to be increasingly important because we’re going to need dynamic and responsive hardware components if we want the data center as a whole also to be dynamic and responsive and draw more or less power during different times of the day, based on the carbon intensity of their energy. In general, you do want to draw more power when you need to, but also draw less when you don’t. So thinking a little about the design strategies, the hardware design strategies, the micro-architecture of the chips will be increasingly important to get energy proportionality.
Stone: A final question for both of you here. We know that computing demands are going to rise, data centers are going to grow dramatically over the next few years. One such forecast from the International Energy Agency is that electricity demand growth will double by 2026, which is just around the corner. It will double again by the end of the decade, depending on which different forecasts that you look at. Are you both optimistic or wary of the future in terms of the demands of AI on the energy system? Again, a lot of efficiencies are going to be happening here, but in terms of just overall demand, that may swallow up the efficiencies. I want to hear what your thoughts are. Dion, let’s start with you.
Harris: Sure, so as I said at the beginning, I think there’s a lot of innovation happening across the data center, across the grid within renewables as a whole. I think that is truly, truly sort of rising to the challenge, if you will, that we’re faced with today. And so what I would say where my optimism lies is in our ability to harness the power of computational processing and AI, to go and tackle some of those other parts of our energy consumption pipeline, if you will. In other words, how can we leverage that compute processing power capabilities of data centers and AI to drive down the emissions of manufacturing, to drive down the emissions of transportation, to drive down the emissions associated with that other 98% of power that’s being consumed and generated? I think that’s a lot where my optimism lies, in that I’m seeing AI being brought to bear, to make climate forecasting and modeling much more efficient, to make material science for developing new batteries and new storage capabilities and technologies much more efficient and improving the overall pace of innovation.
So I do believe that a lot of the work that researchers around the world are doing, and just companies, as well — it’s not just researchers, but companies are doing to help drive innovation through AI, through the computational processing that you get from the data center programs and applications are ultimately going to help drive the innovation that we need to go and tackle the problem and the challenge of renewable energy and tackle the problem and challenge of making a lot of our energy consumption much more efficient and less impactful on the environment.
Lee: I would say that first and foremost, even though I’ve been discussing a lot of the challenges around the energy use in climate, I am a computer scientist, and I am incredibly excited about the future of AI. I think that there are transformative benefits from artificial intelligence, and it’s understandable to think that as we’re developing these AI models, we wouldn’t want to be constrained necessarily by hardware capabilities, by data center capacity, or by energy costs. If a model that we’re training doesn’t work, we might not want to wonder, “Oh, if I’d just put another 20 megawatts of power into it, it could have worked.” I personally feel that there is some room to run, some room to explore with respect to understanding the fundamental capabilities of AI. This may require some additional costs up front that we’re seeing now, today.
I think the second point I would make is that the computer science, computer engineering community has historically been incredibly good at accumulating small, incremental gains in efficiency over many, many years to produce transformative effects on energy efficiency and performance. So I think once data center platforms start to stabilize, once the AI workloads start to become clearer, I think that the target for optimization and efficiency gains will also become clearer. And then there will be a lot of smart minds, a lot of smart people thinking about how to improve efficiency.
And I think finally I’d say that the solution space for energy efficient and sustainable AI is fairly clear. We know that more of it has to be done with renewable energy. We may have to store some of that renewable energy. We may even have to wait for some of that renewable energy to appear at certain times of the day. But that is roughly the solution space for operational carbon. It’s just a matter of figuring out what mix of these solutions we want to deploy and at what cost.
On the embodied side again, we’re understanding the nature of the problem, the nature of semiconductor manufacturing. And we’re beginning to come up with a corresponding solution space there. So I think even though the challenges are fairly significant, we do have a fairly rich toolkit with which we can address these challenges. It’s just a matter of figuring out which combination of these tools we want to use.
Stone: Dion and Ben, thanks very much for talking.
Lee: Thank you.
Harris: Thanks for having me. It was a great discussion.
Stone: Today’s guests have been Dion Harris, Director of Accelerated Computing with Nvidia and Benjamin Lee, a Professor of Electrical Engineering and Computer Science at the University of Pennsylvania.
Dion Harris
Director of Accelerated Computing, NvidiaDion Harris is director of accelerated computing at Nvidia. He focuses on accelerated computing for HPC and AI use cases. Previously, he held various impactful marketing roles in leading data center technology companies such as Dell/EMC and Symantec.
Benjamin Lee
Professor, Electrical and Systems EngineeringBenjamin Lee is a professor of electrical and systems engineering, and of computer and information science, at the University of Pennsylvania. He is a visiting researcher at Google’s Global Infrastructure Group.
Andy Stone
Energy Policy Now Host and ProducerAndy Stone is producer and host of Energy Policy Now, the Kleinman Center’s podcast series. He previously worked in business planning with PJM Interconnection and was a senior energy reporter at Forbes Magazine.