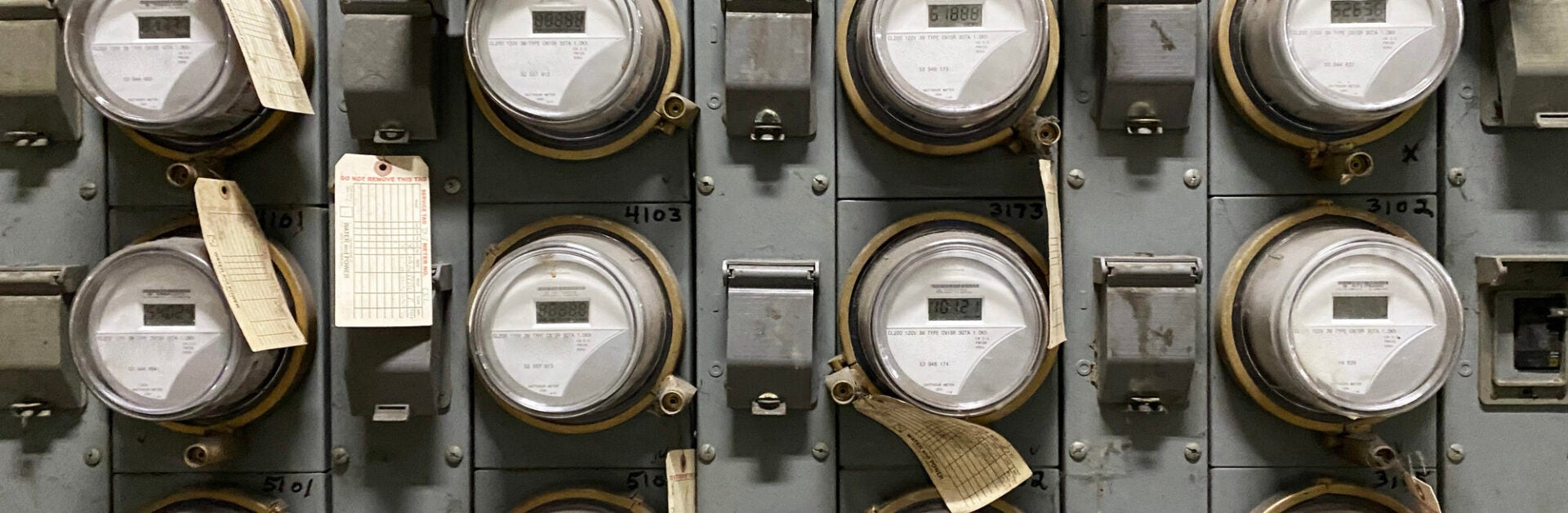
Exploring Electricity Pricing: Are Alternatives to Flat-Rate Plans Worth It?
Time-of-use electricity rates can save consumers money and optimize renewable power. But they can backfire if not carefully designed.
A notable feature of the U.S. electricity system is the disconnect between the cost of generating electricity and the prices most consumers pay for power at any given time. Flat-rate pricing, where consumers pay the same rate for power regardless of demand, discourages efficient electricity use, leading to increased strain on the grid. As concerns about reliability, cost, and climate impacts grow, efficient electricity use is more important than ever.
Kleinman Center Faculty Fellow Arthur van Benthem explores time-varying electricity rates, where prices change with demand, as an alternative to flat rate pricing. Van Benthem, a co-author of new research on the topic explains the potential of time-varying pricing to reduce grid costs and enhance renewable energy. He also explores the potential downside to such rates, which can fail to deliver expected benefits or even backfire if not designed with a focus on simplicity and usability.
Andy Stone: Welcome to the Energy Policy Now podcast from the Kleinman Center for Energy Policy at the University of Pennsylvania. I’m Andy Stone.
A notable feature of the U.S. electricity system is the disconnect between the cost of generating electricity and the prices most consumers pay for power at any given time. Flat rate pricing, where consumers pay the same rate for power, regardless of demand, discourages efficient electricity use, leading to increased strain on the grid. As concerns about the reliability, cost and climate impacts of the electricity system grow, efficient electricity use is more important than ever.
In today’s podcast, we’ll explore alternatives to flat-rate pricing. Specifically we’ll be discussing time-varying electricity rates, where prices change with demand. Today’s guest is Arthur van Benthem, a Faculty Fellow at the Kleinman Center and a co-author of new research on the topic. As Arthur will explain, time-varying pricing has the potential to reduce grid costs and enhance renewable energy; but such pricing can fail to deliver expected benefits or even backfire if rate plans aren’t carefully designed with a focus on simplicity and usability. Arthur, welcome back to the podcast.
Arthur van Benthem: Thank you very much.
Stone: To start us out, could you introduce the disconnect that frequently exists between the cost to generate electricity and the price that consumers actually pay for electricity, or the “flat rate?”
van Benthem: The starting point is that the costs of generating electricity vary significantly hour by hour. If there is low demand, only the cheapest power plants run, and the price in the wholesale market is low. But if demand is high, we need more and ever more expensive power plants to run, which then results in high wholesale market prices. And then the disconnect that you mentioned is that retail consumers don’t see those wholesale prices that vary all the time. They usually just pay a flat price, regardless of when exactly they consume their electricity.
Stone: So the paper that you’ve co-authored has a question as its title, and that title and that question is “How Can We Improve the Efficiency of Electricity Pricing Systems?” How does the flat rate lead to inefficiencies in energy use, and are the inefficiencies that you’re referring to purely economic?
van Benthem: Yes, there are actually a number of inefficiencies that come with flat-rate pricing. First and foremost, flat pricing has very poor incentives to reduce for time shifts that move around throughout the day your electricity demand. When the power grid is under pressure with a lot of expensive, dirty plants running, and also prices are high during a peak hour, you as a consumer still have no incentive to cut back since you don’t actually pay these high prices. So we don’t really get the energy savings when we need them the most.
If you, as a consumer, actually paid a higher price during these peak hours, then you would reduce your consumption, or you would shift it to other parts of the day, say you have an electric car that you can charge at different times. But those other inefficiencies, another consequence of flat pricing is that now we’ve spent a lot of money on having power plants around that are essentially just built to meet these extreme peak demand hours, but they only run during a few hours of the year. That’s very wasteful capital investment, which ultimately hits consumers back through higher retail rates.
Stone: So if we cut peak demand, then as you just mentioned, we have less need for all these power plants, and that presumably would save costs.
van Benthem: That’s right. So you shave off some of the worst peaks throughout the year, and that means that you simply need fewer power plants in your electricity generation system.
Stone: We’re going to be talking about two types of time-varying pricing that expose residential consumers to the real cost of producing electricity. These two pricing models are time-of-use rates and what’s known as “critical peak pricing.” How do they work?
van Benthem: Time-of-use pricing specifies rates or price tiers for different hours of the day, say peak versus off-peak or for different days of the week, or say weekend versus weekdays, or even seasons. You might have slightly higher summer prices than winter prices. So it’s a set price that’s set in advance, and you as a customer know when different price tiers apply.
Now critical peak pricing is a little bit different, so that adds a cost premium to very specific blocks of time. We usually call them “events.” There are only a few of them that a utility can call during a year, and those events will be called when the power system is expected to be near-capacity based on the current price that we see in a market or the price that we expect, say, one day before.
So then what happens is that you as a customer typically get a text message, say a day before the utility wants to call an event, and it’s going to warn you, “Hey, tomorrow your price is going to be really high between 4:00 and 6:00 p.m.” So you have a little bit of time to plan ahead.
Stone: Your research compares these two pricing solutions against the ideal, which is what’s called “real-time pricing.” And real-time pricing, again, as the ideal, would deliver the greatest savings, but it hasn’t really proven to be workable for consumers in a practical sense. How does it work, and why has it not really worked out?
van Benthem: Yes, I think you’ve summarized it very nicely. There’s something very nice about real-time pricing that I will talk about, but also there are a lot of tricky, tricky issues. What real-time pricing does is it would let consumers face the true cost of electricity generation at every point in time, in addition to the transmission and distribution costs that consumers would also pay. And therefore, it would always align the true cost of generating and bringing the electricity to your household at every single point in time. So you would totally get rid of any sort of mispricing and inefficiency.
That’s why, in some sense, it might be considered ideal. Now the issue is that it does expose customers to potentially quite high price risks. For example, Winter Storm Uri in Texas in 2021, where wholesale market prices skyrocketed — they went into the thousands of dollars in a market where typical prices are, say, in the 30s or 40s. That became front page news because a small group of consumers had actually opted into real-time pricing, and then when those hours with extreme prices happened, they paid orders of magnitude more for their electricity consumption. They were not fully aware, and in the most extreme cases, people faced electricity bills, a monthly bill, in the tens of thousands of dollars. That’s sort of the worst-case scenario, right?
So the issue is that with the efficiency benefits from real-time pricing really hinge on a very important premise, which is that people can and do respond to changing prices. You need to be really on top of what’s happening in the power market to make sure that you avoid prices when they’re high, and you take advantage of prices when they’re low. So it takes time and effort and experience to adjust your power usage, and realistically, a lot of people wouldn’t want to barter with that, unless there’s some smart home automation software that just does it for you.
Stone: That’s really beyond the sophistication and engagement with the electricity system that most people would really have.
van Benthem: Yes, if you had to do this manually, it seems a lot to ask from consumers, to continuously and actively tweak their electricity demand. I think that’s the reason why real-time pricing has, so far, stalled for the most part.
Stone: Given that the challenges involved with real-time pricing, particularly for residential consumers who aren’t really set up to get engaged with the electricity system in pricing and in real time, we have time-of-use rates and critical peak pricing which are sort of a second-best but more workable solution. But maybe counter-intuitively, the simple programs, the simple time-of-use programs that are being designed or that we’re going to talk about here deliver more reliable savings than more complex and sophisticated programs. And this is really important in designing these programs. Can you talk about why your research has shown that these simple programs are more efficient? What is this the case?
van Benthem: Yes, absolutely. That was perhaps a surprise for us, too. When you say “simple programs,” we just think about a very simple time-of-use system where you just have, for example, a peak price from late afternoon, early evenings and an off-peak price for the rest of the day. A complex program could be something where the price is set for every hour of the day having a different price, for example. Now the issue really is that utilities that use time-of-use programs will need to predict peak and off-peak prices for the current year, based on something like average prices during these peak periods in previous years. That’s the information they have. But of course, this year may look quite different from the past three years, and what we tend to find is that price differentials between peak and off-peak periods within a day, or something else that’s simple — weekend versus weekday pricing — those price differentials tend to be fairly steady over time. But the more pricing categories you introduce, like different tiers for each hour of the day and of the week and what have you, the less likely it becomes that the historical price pattern is going to be a good match going forward. And that’s what’s called “over-fitting.” That pricing system might have worked well in the past, but actually it does worse in the future.
What I find interesting is that when you look at what some utilities are doing — one example of that which comes to mind is San Diego Gas & Electric — is that they’re making these time-of-use systems more and more sophisticated by having more and more different pricing tiers. But our findings suggest that that might actually backfire, and it’s not clear at all that that’s going to deliver more benefits than just sticking with a very simple policy.
Stone: So basically the more granular you get, having more pricing periods on the hour or whatever it may be, the more opportunity you have for that very specific timeframe to differ from the historic patterns that the programs might be based upon.
van Benthem: Absolutely. If you take this to the extreme, and you take 3:00 pm on August 22nd of last year, which had a very high price, say, because there was very high temperature. If you’re going to use that to predict the same hour, the same day next year, there’s no reason why that specific hour would have such a high price, and the price would be completely misaligned. So that’s sort of the tradeoff you face with over-fitting. And that’s actually a common finding across forecasts in general, statistically — not just for electricity pricing.
Stone: I’m curious. What data did you look into or model to come up with these conclusions on the importance of simplicity?
van Benthem: There’s really great data on electricity markets. The seven largest wholesale markets in the U.S. published their hourly pricing at every different node or location in the system for sometimes over 15 years, so there is extremely granular data on what price happened in the wholesale market in each different market, each different location, for many years in the past.
Stone: Okay, so how might simplicity that we’ve just talked about, and another key criterion that the paper points out, which is predictability — How might these two be designed into the pricing options we discussed? And I wonder if you could talk about that predictability element?
van Benthem: Yes, so briefly you mentioned simplicity, right? We’ve talked about this a bit now. I think that’s where it might be used in a very simple way, which is just don’t use more than, say, four pricing tiers. We find using our machine-learning algorithms, that setting two or at best four, something like peak versus off-peak, and then maybe you will set different peak versus off-peak prices for weekends, and then separate from weekdays. That’s about as good as it gets. Once you go more granular, it tends to backfire.
Predictability is a different issue. Let’s take the extreme of real-time pricing. There you introduce a really large amount of bill uncertainty, right? Prices vary all the time. There’s not much predictability, and some months see much higher average prices in the market, and your bill might change much more abruptly from month-to-month than what you’re used to now. So if you move to a system like that, you give up some predictability, but you gain efficiency. And that’s the key tradeoff here.
With family use pricing, you get much more predictable pricing. Actually you know your peak and off-peak prices and during which hours they apply far in advance. But then also, the efficiency gains are more limited. So there’s always this tradeoff between predictability, which is good for consumers, but it comes at the expense of overall efficiency or cost savings.
Stone: It sounds like if you get more efficiency, you actually put consumers potentially more at risk for suffering price shocks.
van Benthem: Absolutely. It’s a pure tradeoff.
Stone: Okay. I’m just curious. What are the potential savings with time-of-use rates in critical peak pricing, versus the ideal, which is, again, the real-time rate?
van Benthem: We estimate that time-of-use pricing systems and critical peak pricing can each restore, say on average over various years and across different power markets in the U.S., about 10% of the efficiency gain that you could achieve if you moved to full real-time pricing. If you actually decided as a utility to do both — so you have some form of time-of-use pricing, and then you add to that, say 20 critical peak events that you can call — those gains roughly add up to 20%.
Now the question here that I’ve been debating with my colleagues quite a bit is: Is that big or small? I think that depends on how you think about it. On one hand, none of these policies clearly come anywhere close to the cost savings that you could achieve if you expose everyone to real-time pricing. But the other thought is that these policies, time-of use and critical peak pricing, are pretty easy to implement. In fact, they have been implemented. They have proven possible, and you still get a 20% gain that translates to quite a few millions of dollars every year. So I think from that perspective, it sounds like a really good idea to at least have some form of smartly designed time-of-use and critical peak pricing.
Stone: Let me ask you another question here. It’s something that actually came up also in the research that I found very interesting. It’s the fact that these pricing programs that we’re talking about here, where consumers respond to demand actually are very good for maximizing the potential of renewable power. Yet at the same time as we get more renewable power onto the grid, the complexity of these pricing schemes becomes more real, more extreme. So renewables actually make the implementation of these programs a little bit more difficult. Is that right?
van Benthem: Well, what renewables in the grid are going to do is add more volatility to the wholesale market pricing. There might be hours when there’s lots of wind, so there’s so much supply that prices are, say, around zero. But there might also be days where wind is under-delivering, and it’s evening, say, there’s no solar where the grid would see a really, really high price. So in that sense, it’s even more important that consumers face the right price signals. But it also means that bill uncertainty is going to be even larger. And that’s the critical challenge that we need to deal with.
The environmental benefit, though, of real-time pricing would be that it gives consumers incentives to switch consumption to low-price hours which tend to be hours with cleaner electricity. So in addition to the pure cost savings from a grid perspective, it’s also very likely that moving a little closer towards real-time pricing or adding more time-varying elements to pricing will reduce carbon emissions.
Stone: Arthur, you talked a few minutes ago about the example of San Diego Gas & Electric, which has offered time-of-use pricing to its customers, and you also mentioned that their time-of-use structure is rather complex. To what extent, beyond San Diego Gas & Electric throughout this country, have time-of-use and critical peak pricing programs actually been offered to residential consumers? And to what extent generally have the priorities of simplicity and predictability been adhered to in designing these programs?
van Benthem: Benthem: Time-of-use pricing is very common. A very large number of utilities offer TOU plans to their customers. Critical peak pricing programs are much more rare. They definitely happen across the country, but it’s far less likely that your utility would offer you a CPP plan than a TOU plan. I think utilities so far have stuck with relatively simple time-of-use rates, so in that sense, simplicity has been present, although there is a current trend where you see time-of-use systems becoming more “sophisticated,” which is directly related to the main point of our paper. That may make sense at the service but actually runs into the danger of overfitting. So our research findings don’t necessarily suggest that that is a productive direction to go into.
Stone: As you briefly noted earlier, technology seems pretty important. Smart appliances, for example, might be able to capture utility price signals and respond to them, then in turn take advantage of time-of-use rates and critical peak pricing — and even real-time pricing. Could you talk about the enabling potential of technology, and maybe technology would allow real-time pricing sometime in the future to be viable?
van Benthem: Yes, that’s a really good point, Andy. The last thing I personally would want to do is sit in my office now, talk to you, and then pause at the pressing buttons in an app and adjust my lighting at home while we’re talking now. That seems really, really cumbersome. So I think that smart appliances and home automation are absolutely crucial for the long-term success of real-time pricing. It takes the thinking about it out of the equation. And honestly, it’s also going to be much better than either you or me at deciding when to run our appliances and to minimize our power bills.
So this is going to be, if implemented successfully, a fairly painless way to avoid being surprised by price spikes. The home automation would immediately reduce consumption dramatically if there were ever a Texas-style price fight. And then as an added bonus, it’s going to contribute to grid stability by shaving off peaks. It’s going to make it easier to integrate renewables. I’m extremely, extremely excited about the potential of home automation and smart technology to make our electricity system more efficient.
Stone: Let me ask you a final question here. Obviously we’re a policy podcast, so I want to ask you: To what extent are policy-makers aware of the potential for the pricing programs that we’ve talked about to increase efficient use of the electric grid? What’s out there in terms of moving these policies forward in the near future?
van Benthem: Policy-makers are certainly aware that retail pricing is not efficient. I don’t think that’s a hidden problem to them. I think it’s more that kind of political backlashing concern understandably from customers about these varying bills has stalled any sort of meaningful progress towards real-time pricing. So what I would say is that either you can accept that and say, “Okay, we have to stick with time-of-use and critical peak pricing structures,” and then as we discussed, there are at least a couple of things you could do to make them a little better. So keep it simple for time-of-use. And for critical peak pricing, what’s absolutely crucial is that if you call these events, you need to set a critical peak price with timely information. So say you used — the day you had a price. The price that you have at a forum are good a day before you call the event, and apply that to that specific event. Why is that important? Because not every critical peak event is borne equal. Some are extreme, extreme events. Some are extreme but less extreme events. And the price that actually materializes in the wholesale market might still be very different.
So what utilities sometimes do is they set a single critical peak price based on information from previous years and apply that to all their peak events. But there’s very little connection between that specific peak event and that price that they chose based on previous years. We find that if you do it that way, there’s hardly any efficiency benefit.
So those are little things you can do to improve upon the current systems. But what I would actually like the utilities to do is think a little more out of the box and move to a system that you could call something like “limited real-time pricing.” So this is real-time pricing with a price gap. The real concern, of course, that everyone has about real-time pricing is, “What if wholesale prices shoot up to $1,000 per megawatt hour or even more?” But in a vast, vast majority of hours, wholesale prices fluctuate somewhere between, say, zero and $70 per megawatt hour — nothing too extreme.
Now our research also finds that if you put customers on real-time pricing, as long as the price can never exceed some pretty restrictive ceilings, say $70 per megawatt hour, you can still capture about two-thirds of the efficiency gains from full real-time pricing. So you don’t get the gains when prices go too crazy, but in all the other hours, prices and costs are perfectly aligned. And this is not going to lead to the crazy fluctuations in power bills. I think this is the kind of pricing system that utilities might seriously consider swapping out TOU for, and it might actually deliver much better cost savings.
Stone: Arthur, thanks very much for talking.
van Benthem: Sure, my pleasure. Thanks for having me.
Stone: Today’s guest has been Arthur van Benthem, a Faculty Fellow with the Kleinman Center for Energy Policy.
Arthur van Benthem
Associate Professor of Business Economics and Public PolicyArthur van Benthem is an expert in environmental and energy economics, exploring the economic efficiency of energy policy. He is a faculty fellow at the Kleinman Center and an associate professor of Business Economics and Public Policy at Wharton.
Andy Stone
Energy Policy Now Host and ProducerAndy Stone is producer and host of Energy Policy Now, the Kleinman Center’s podcast series. He previously worked in business planning with PJM Interconnection and was a senior energy reporter at Forbes Magazine.