
The Changing Landscape of IAMs and Opportunities for Improved Climate Policy
Discover how new, dynamic Integrated Assessment Models (IAMs) are empowering policymakers to design tailored, region-specific climate policies with greater transparency and precision.
In a recent article (forthcoming at the Annual Reviews of Economics), we review the recent progress of structural Integrated Assessment Models (IAMs), which have evolved significantly in recent years thanks to advances in machine learning and computational power. An Integrated Assessment Model is a computational tool that combines knowledge from multiple disciplines, such as economics, environmental science, and energy systems, to assess the impacts of climate change and the effectiveness of policies for addressing it. Improvements to these models offer substantial benefits for policymakers at national, regional, and global levels, providing more accurate, dynamic tools for quantifying the economic effects of climate change.
Policymakers often use or refer to IAMs in reports like the IPCC’s Sixth Assessment to quantify risks such as species loss, food insecurity, and the effects of policy on global emissions. National governments also rely on IAMs to assess the impact of climate initiatives like the Inflation Reduction Act in the U.S., helping them set realistic targets for emissions reductions and determine the social cost of carbon (SCC).
How Do IAMs Work?
Figure 1 provides a stylized representation of an Integrated Assessment Model (IAM), showing the interaction between the economy, climate, and damage modules. It highlights how economic activity generates CO₂ emissions, which affect global mean temperatures (𝑇𝐴𝑇) and lead to economic damages. Uncertainty is represented in each module, as indicated by the sigma (σ) symbol, reflecting the unpredictable nature of emissions, temperature changes, and their impacts.
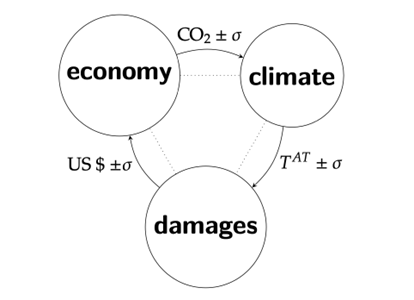
These models are inherently complex due to the uncertainties and intricacies of the relationships between climate and economic systems. They require vast amounts of data for estimation, are forward-looking, and are dynamic and evolving over time. This makes the quantification of uncertainties particularly challenging.
A New Generation of IAMs
The evolution of Dynamic IAMs has broad applications for macroeconomics and policy. Dynamic IAMs can focus on global climate models or can incorporate higher spatial resolution to analyze regional temperatures or damages. IAMs can also vary according to the level of uncertainty they incorporate and whether they focus on a single representative agent or several heterogeneous agents.
Updated models incorporate new capabilities empowered by enhanced computational power and data improvements. Enhancements to IAMs include:
- Better Standards and Transparency: New models, like CDICE, offer greater transparency by using standardized benchmark data from climate science, making them more accessible and reliable.
- Enhanced Accuracy: Improvements in machine learning, such as pattern recognition algorithms, and improvements to damage functions, which quantify economic loss resulting from climate change, enable IAMs to better account for uncertainties and non-linearities, such as tipping points in the climate system. These serve to provide better-estimated policies and methods for checking uncertainty.
- Spatial Resolution: Deep learning and other computational advancements now enable more detailed regional projections, helping policymakers tailor strategies to specific geographic areas and reduce the risks of one-size-fits-all solutions.
While IAMs are still imperfect, these advances can significantly enhance policy decision-making compared to the status quo.
Applying IAM Improvements to Policy
For policymakers, these advancements open new opportunities to design and evaluate climate strategies more effectively.
- Determining the Social Cost of Carbon (SCC): The Social Cost of Carbon (SCC) estimates the economic damages from emitting one additional ton of CO₂, helping to inform climate policies like carbon pricing. New IAMs provide more robust SCC estimates by considering distributional effects across income, generations, and regions, improving policy reliability. Policymakers can choose from several methods for calculating SCC, including traditional approaches that are limited in their ability to incorporate uncertainty and may underestimate risks or methods that incorporate “deep uncertainty” for stronger precautionary measures. Within these methods, a model can also account for factors such as intergenerational equity to better ensure fair distribution of costs and benefits across generations.
- Designing Region-Specific Climate Policies: With improved spatial resolution, policymakers can create region-specific adaptation and mitigation plans. This can ensure that climate policies are more equitable, as the impacts of climate change vary widely between regions. This has a wide range of applications but may be particularly useful for policymakers in a global setting, such as the Conference of the Parties (COP), to ensure that the model considers regional differences and realities to reach equitable and cooperative agreements.
- Creating Politically Feasible Solutions: Improved IAMs can help policymakers avoid the “green paradox,” where environmental policies inadvertently worsen emissions in the short term. By anticipating ex-post errors through more Stochastic models, IAMs enable policymakers to set more realistic and politically viable targets for a net-zero transition. Overall, improved and more dynamic IAMs can provide more options for policymakers based on the political realities of that time. For example, policymakers may seek to compare inflation-resistant carbon tax strategies to socially and monetarily harmonized carbon taxes.
- Integrating Energy Market Dynamics: By incorporating data from energy markets, IAMs can offer insights into the costs and benefits of transitioning from fossil fuels to renewable energy. By integrating energy prices, industrial trends, and technology forecasts, these models help optimize climate policies and identify the most cost-effective paths for reducing emissions.
Recent IAMs have revealed that achieving deep emissions cuts may require repurposing or stranding existing industrial capital, such as power plants and steel mills. This is due to the high costs of emissions reductions and capital inertia—the difficulty of rapidly shifting away from entrenched, carbon-intensive infrastructure. By using IAMs to analyze energy transitions, policymakers can better understand when and where to retire or retrofit existing assets and allocate resources more effectively to support clean energy technologies.
A New Era for Climate Policy
The recent advancements in IAMs have significantly improved their utility for climate policymaking. These tools now offer greater accuracy, transparency, and flexibility, allowing governments to design climate policies that have the potential to be more effective and fairer. By leveraging these improvements, policymakers can take more confident, data-driven action toward decarbonization.
Jesús Fernández-Villaverde
Professor of EconomicsJesús Fernández-Villaverde is a professor of economics and research associate for the National Bureau of Economic Research (NBER) and Penn’s Population Studies Center, and a Research Affiliate for the Centre for Economic Policy Research.
Kayla Main
Kleinman Center Research AssistantKayla Main is a Research Assistant at the Kleinman Center. Through the Kleinman Center, she also works with Energia, an international network supporting gender equity and sustainable energy progress, and their partners, such as Solar Sister.