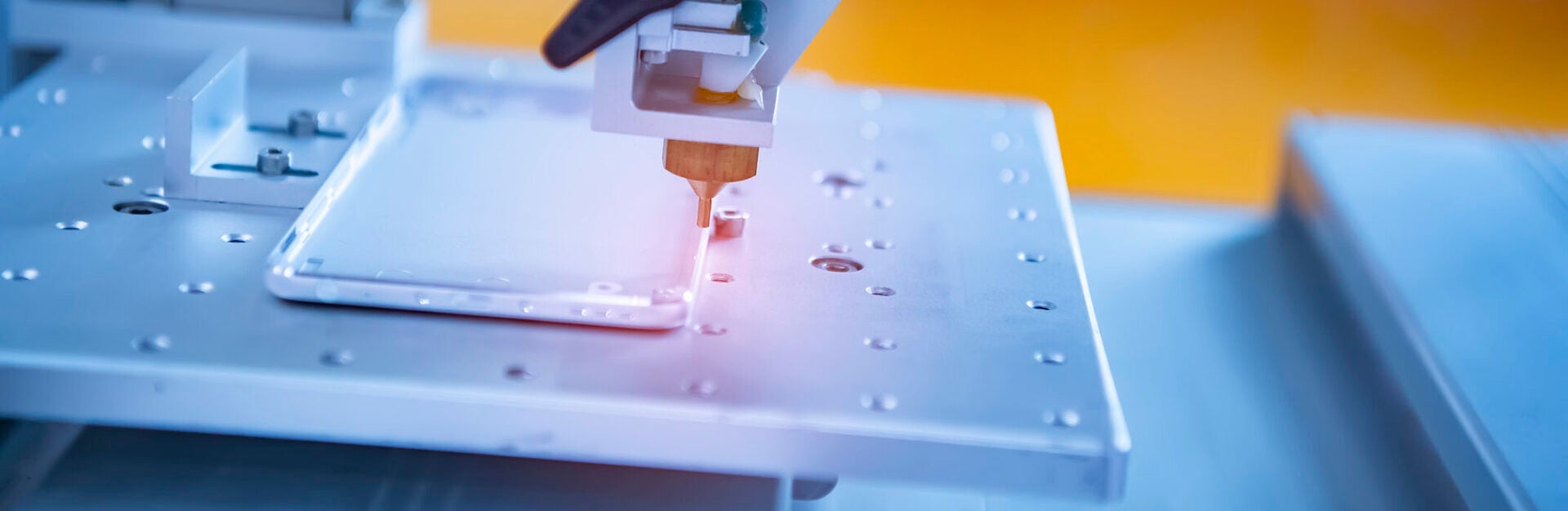
Grace Under Pressure: How AI Can Help Us Design the Next Generation of Smart Separations Membranes
AI can provide a new approach for using polymers for carbon emissions reductions, propelling us closer to victory in the fight against climate change.
This blog is the written winner of our 2023 Blog Competition.
Separating gas mixtures has been a cornerstone of process engineering for as long as the field has been around. Purifying oxygen from nitrogen for medical and industrial applications, upgrading renewable natural gas before it enters our homes, and as of late, separating carbon dioxide from our atmosphere at parts-per-million concentrations are all areas of critical importance to keep the global economy functioning.
Despite their simplicity, though, traditional separations processes like distillation or other thermally driven methods have a big drawback: their carbon intensity. In 2016, separations made up half of the industrial energy sector in the United States and more than 10% of our total energy consumption. The amount of fossil fuels needed to purify these mixtures is immense. In the case of CO2 capture from our atmosphere to fight climate change, this process (if fueled by fossils) could defeat the engineering purpose we’re trying to achieve.
Reducing the emissions that come from separations presents a crucial challenge in the struggle against climate change, and one that we are on an increasingly shorter timescale for implementing. An attractive avenue for doing this is via the use of membrane-based separations: with your gas mixture on one side of a thin membrane at high pressure, you can remove a purified product stream on the other side by filtering gas through its pores.
Think of it like sifting sand through a screen at the beach to remove the rocks—only in this case, the screen holes are fractions of nanometers in size. By better understanding their properties, scientists can design new membranes from long, spaghetti-like polymer molecules that are optimized for performance at scale.
Making designer polymers that are specific enough for the task at hand but still allow enough throughput to be used effectively is an exciting frontier in materials research. New classes of molecules like ladder polymers, with rigid structures that contort into networks of continuous nanopores, have shown themselves to be incredible candidates for some of these applications. But the path to improving these and pushing them to the next level of performance is not always clear.
Because the design spaces for polymers are so vast, exploring them entirely is nearly impossible. A new approach finding the best candidate polymers is to prescreen them with another tool that’s a hot-button topic: artificial intelligence. Right now, AI has been employed in many areas of scientific research, from predicting protein folding structures, to understanding the dynamics of molecular glass, and even optimizing agricultural systems to improve large-scale crop yields.
Identifying the patterns in massive amounts of data allows us to bridge gaps between theory and experiment. AI helps us find relationships not yet understood and can even help identify the missing links.
Using existing databases of polymer properties in literature, scientists can construct neural networks to predict polymers that have never been made, which might have the properties needed for a particular application. From there, detailed atomistic-scale simulations can be used to model the most promising molecules and then take them to another level of screening. These computational techniques have been utilized for decades now but are themselves expensive due to the carbon footprint of scientific computing.
Narrowing down our choices makes using this level of detail a more reasonable option. The best of the best polymers will be the ones that make it to the final, and most expensive stage: real-life synthesis and testing.
Taking this machine learning-aided pipeline approach not only allows scientists to hone in on their choice of materials much faster, but also saves valuable time and resources in manufacturing the next generation of high-performance materials. Attacking not only gas separations processes, but other separations that could help turn the tide of the global climate crisis—like the removal of rare earth elements from their ores or extracting uranium from seawater—means that the importance of these novel polymers can hardly be overstated.
Policymakers and scientific agencies should consider just how incredible the potential for using AI-accelerated science is in these cases, and how more funding for the next generation of autonomous experimentation in materials research could lead to the breakthroughs that help win our collective fight against the global climate crisis. Augmenting scientists’ existing tools with the power of big data harnessed through machine learning means that the scope of the problems they can address can be increased to match the existential climate threat that we are facing.
Sam Layding
PhD Candidate, Department of Chemical and Biomolecular EngineeringSam Layding is a PhD candidate in the Department of Chemical and Biomolecular Engineering and a 2023 NSF Research Trainee Fellow for Interdisciplinary Training in Data Driven Soft Materials Research and Science Policy.